- Amplifying Cognition with Ross Dawson
- Posts
- GenAI and strategic foresight, metacognitive efficiency, AI for human agency, and more
GenAI and strategic foresight, metacognitive efficiency, AI for human agency, and more
As more and more artificial intelligence is entering into the world, more and more emotional intelligence must enter into leadership. - Amit Ray
Building an AI Roadmap, LLMs, and collective intelligence
I have just arrived in the US. The first half of this week I will be at LinkedIn’s Santa Barbara studios to record a LinkedIn Learning course on Building an AI Roadmap, after which I’ll head to San Francisco to catch up with the forefront of AI.
This week’s podcast conversation is with Jason Burton, the lead author of a prominent new article in Nature on “How Large Language Models Shape Collective Intelligence”. This is a critically important topic; I recommend listening to Jason’s insights in episode.
And below my weekly essay looks at 4 different approaches to content curation.
Be well!
Ross
📖In this issue
Metacognitive efficiency for better Human-AI collaboration
Increasing human learning and agency with AI
A meta-analysis of Human-AI collaboration and future directions
Generative AI and strategic foresight
Jason Burton on LLMs and collective intelligence, algorithmic amplification, AI in deliberative processes, and decentralized networks
Ideas to execution faster, cheaper, better
🧠🤖Humans + AI
Metacognitive efficiency for better Human-AI collaboration
An interesting new paper focuses on “metacognitive efficiency”—the ability to self-assess accurately—in optimizing human-AI collaboration. High-performing users particularly benefit from this skill, as it helps them determine when to rely on AI advice, leading to better decision quality. For lower-performing users, AI advice reliably enhances outcomes, but high-performers gain only if they have strong metacognitive skills.
Know Thyself: The Relationship between Metacognition and Human-AI Collaboration
Key insights from paper
Increasing human learning and agency with AI
I’m a big fan of a new paper that proposes that Human-AI interaction should apply "reciprocal algorithmic output" that increases human agency and learning, even when the AI is removed.
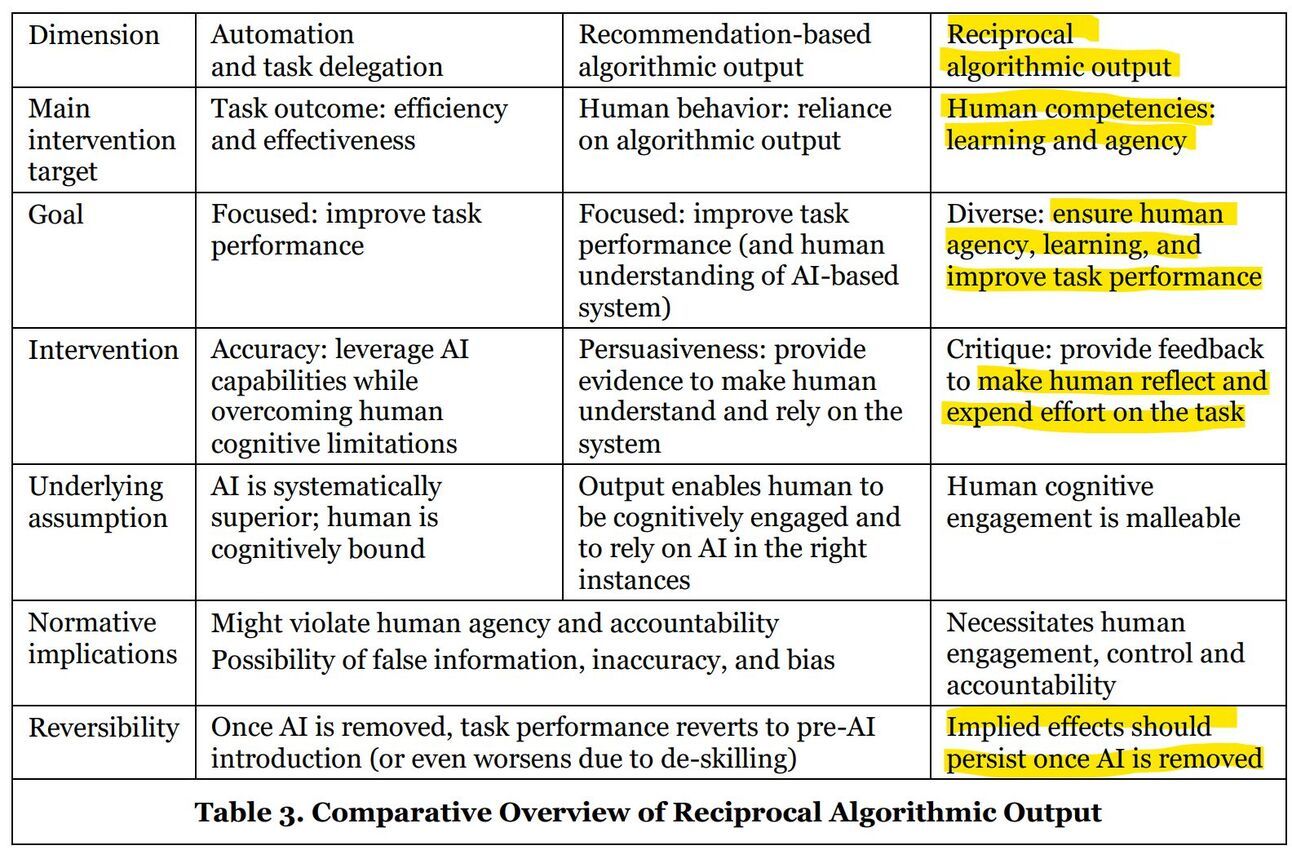
A meta-analysis of Human-AI collaboration and future directions
A meta-review in Nature Human Behavior doesn’t paint a bright picture of Human-AI collaboration: “We found that, on average, human–AI combinations performed significantly worse than the best of humans or AI alone… When humans outperformed AI alone, we found performance gains in the combination, but when AI outperformed humans alone, we found losses.“
However this was across all tasks surveyed. In creative tasks there are positive synergies which the authors believe could be increased. They also point to the potential for innovative co-creative processes, and strong limitations to the research they surveyed. The paper is interesting mainly for its guidance in better future research on the topic.
Generative AI for strategic foresight
A report from IF Foresight: “his report illustrates how GenAI’s integration into Futures Studies and Strategic Foresight is more than an advancement; it’s a paradigm shift that will deeply impact data analysis, scenario generation, strategic decision-making, and creative processes—fundamentally transforming our approach to anticipating and shaping the future. “
It’s a vital topic that we will be covering more in coming months.
🎙️This week’s podcast episode
Jason Burton on LLMs and collective intelligence, algorithmic amplification, AI in deliberative processes, and decentralized networks |
Why you should listen
Jason Burton is lead author of the recent much discussed Nature paper "How Large Language Models Can Reshape Collective Intelligence". In this conversation we dive deep into the paper's findings, as well as other aspects of Jason's fascinating work, including algorithmic amplification, how the network structures of social media shapes its impact, and how AI can play a role in democratic and deliberative processes.
💡Reflections
4 different types of content curation for a fast-moving world
A couple of days ago I did a LinkedIn post on the report “How Generative AI is Transforming Strategic Foresight” shared above in the newsletter.
My friend Sami Makalainen had a look and was pretty critical of the report, both in format and in pointing to some deficiencies, such as the way it framed explainable AI.
This made me reflect on good practices in curation, which is central to how people get information and build their profiles.
Creating value with curation
I have long been a curator, first on my blog (since 2002) and then as my primary way of using Twitter (from 2008).
Finding, selecting, and sharing content creates massive value. First, in helping people find what is useful amid an increasing proliferation of information of highly variable quality.
And also in encouraging you to seek out the best, to understand what you’re sharing, and to become visible in a world of overload.
In my book Thriving on Overload I describe how curation can play a central role in effective information filtering.
Types of curated sharing
The primary dimension Sami and I discussed was whether to provide critiques of what you share. That points to a few different approaches to sharing content.
Link only. Sharing only a link is lazy. It says nothing about who you think will find it interesting or useful, whether you think it is brilliant or just OK, or sharing for a range of other reasons.
Link with comments. This can be far more useful, giving people an indication of why they should follow the link and what they will find when they get there.
Link with main insights. This is the approach I have been mainly using on LinkedIn lately, sharing what I find most interesting and useful from what I see, saying why I think it is important and summarizing the most striking insights in the linked content, or simply pointing to an interesting implcation. This means that people can get value from the post itself, and follow the link if they want more detail.
However people can interpret this as a full endorsement of the source material. Sharing interesting insights doesn mean that the methodologies are good or that other aspects of the content are even correct.
Link with full critique and analysis. This can provide the most value, in not only sharing what is most useful, but also analyzing the strengths and deficiiencies of the content so that people can be guided in getting the most value from the original content.
This is a lot of work, so limits how much you can share. Also, in a low attention span world many people will not go to the source, and the additional context won’t be useful to them.
There are of course many other dimensions and variables to how you share content. But this framing does help think about how you can go about being a good curator.
Thanks for reading!
Ross Dawson and team